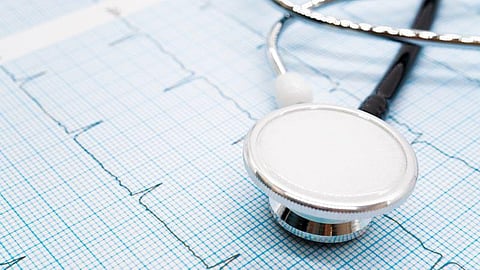
TUESDAY, May 14, 2024 (HealthDay News) -- A deep learning model using transthoracic echocardiograms (TTEs) can predict patients with active or occult atrial fibrillation (AF), according to a study published online April 13 in npj Digital Medicine.
Neal Yuan, M.D., from the University of California in San Francisco, and colleagues created a two-stage deep learning algorithm using a video-based convolutional neural network model that distinguished whether TTEs were in sinus rhythm or AF and then predicted which of the TTEs in sinus rhythm occurred in patients who had recently experienced AF. The model was trained on 111,319 TTE videos.
The researchers found that in a held-out test cohort, the model was able to differentiate TTEs in AF from those in sinus rhythm with high accuracy (area under the receiver operating characteristic curve [AUC], 0.96; area under the precision-recall curve [AUPRC], 0.91). The model predicted the presence of concurrent paroxysmal AF among TTEs in sinus rhythm (AUC, 0.74; AUPRC, 0.19). In an external cohort of 10,203 TTEs, model discrimination remained similar (AUC, 0.69; AUPRC, 0.34). Performance was similar among patients who were women, were older than 65 years, or had a CHA2DS2VASc score ≥2. Model performance was better than use of clinical risk factors, TTE measurements, left atrial size, or CHA2DS2VASc (AUCs, 0.64, 0.64, 0.63, and 0.61, respectively). Performance was better for an ensemble model in a cohort subset combining the TTE model with an electrocardiogram (ECG) deep learning model than the ECG model alone (AUC, 0.81 versus 0.79).
"Deep learning by TTE may offer additional opportunities to guide patient screening for occult atrial fibrillation by identifying patients who may benefit from more intensive monitoring," the authors write.