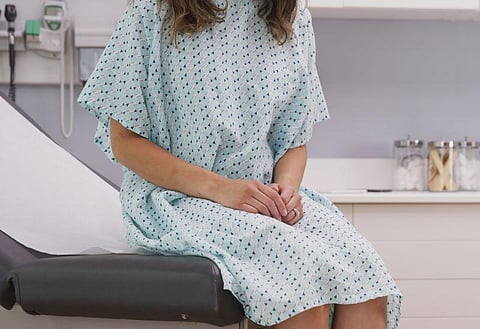
WEDNESDAY, Dec. 27, 2023 (HealthDay News) -- Machine learning (ML) model performance for diagnosing bacterial vaginosis (BV) varies among ethnic groups, according to a study published online Nov. 17 in npj Digital Medicine.
Cameron Celeste, from the University of Florida in Gainesville, and colleagues examined the ability of four ML algorithms to diagnose BV. Fairness in the prediction of asymptomatic BV using 16S rRNA sequencing data from Asian, Black, Hispanic, and White women was investigated.
The researchers observed variation in general purpose ML model performances based on ethnicity. Models performed least effectively for Hispanic and Asian women when evaluating the metric of a false-positive (FPR) or false-negative rate (FNR). In general, models had the highest and lowest performance for White and Asian women, respectively.
"Here, we show that several supervised learning models perform differently for ethnic groups by assessing commonly used metrics, such as balanced accuracy and average precision, as well as more clinically relevant metrics, such as FPR and FNR, in a cohort of women with asymptomatic BV," the authors write. "The results provide evidence that there is a discrepancy in model performance between ethnicities."